Revolutionizing Data Annotation with Bounding Box Tools
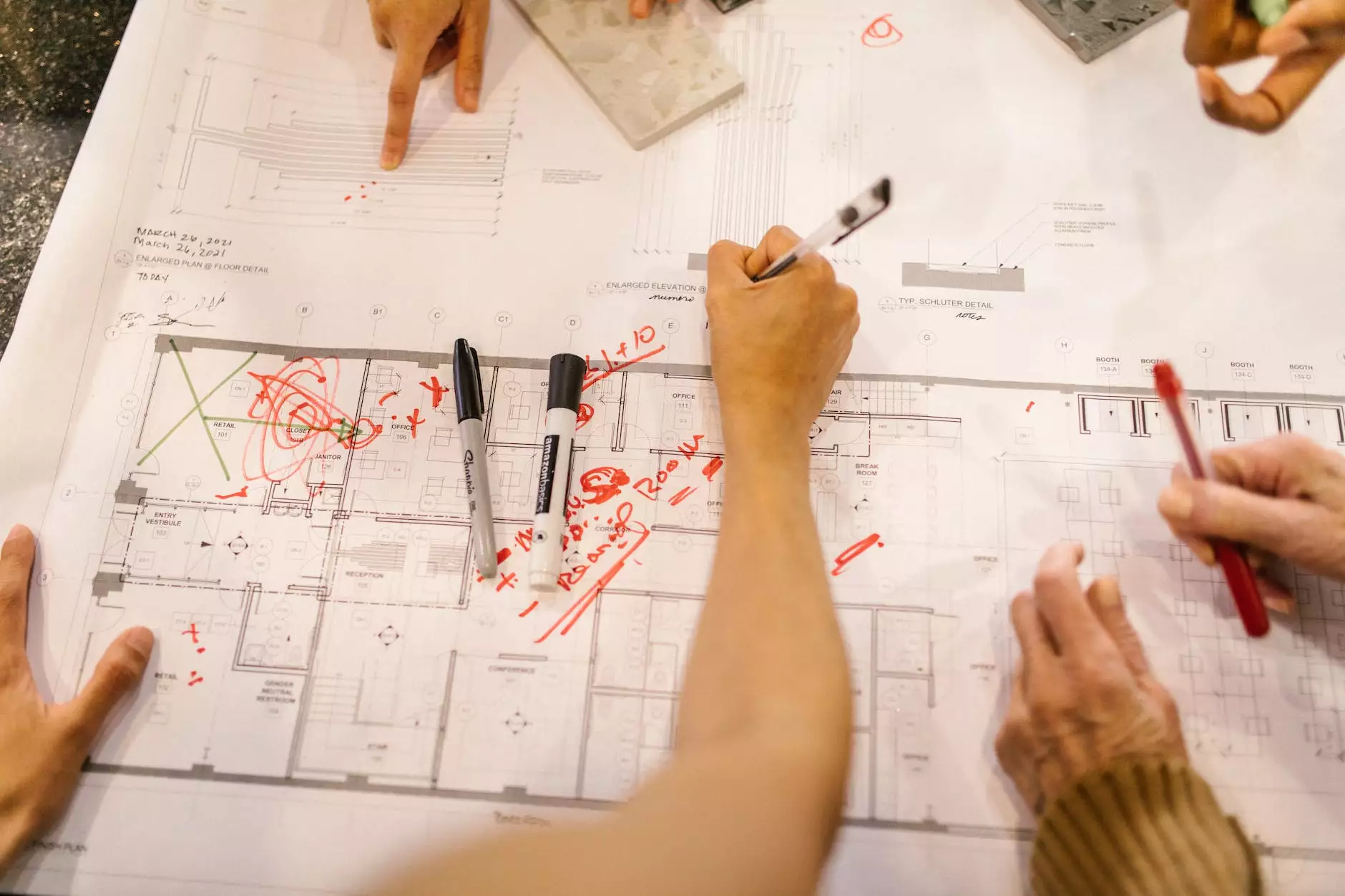
In today's data-driven world, businesses are constantly seeking innovative ways to extract actionable insights from vast amounts of data. One of the most essential aspects of this process is data annotation, which ensures that machine learning models are trained correctly to make accurate predictions. Among the various methods of data annotation, the use of bounding box tools has emerged as a critical component. This article delves into the importance of bounding box data annotation tools, their functionalities, advantages, and how they can transform your organization’s data processing capabilities.
Understanding the Basics of Data Annotation
Data annotation is the process of labeling data to provide context for machine learning algorithms. This process includes various tasks such as:
- Image Annotation: Tagging objects in images for computer vision tasks.
- Text Annotation: Identifying entities and sentiments in text data.
- Audio Annotation: Transcribing audio files or segmenting audio tracks.
The quality and accuracy of the annotated data are paramount, as they directly influence the performance of machine learning models. Among these annotation techniques, bounding box annotation is particularly significant for image-related tasks, where it is crucial to identify and locate objects within images effectively.
What is a Bounding Box?
A bounding box is a rectangular box that is drawn around the objects in an image to define their position and shape. It is a fundamental concept in computer vision, particularly in tasks such as object detection, image segmentation, and localization. By utilizing a bounding box, machines can be trained to recognize various objects and their boundaries, enabling more advanced functionalities like autonomous driving and facial recognition.
Key Features of Bounding Box Annotation Tools
When considering a data annotation platform that utilizes bounding box tools, it is critical to understand the features that make these platforms effective:
- Intuitive User Interface: A user-friendly interface enhances the productivity of annotators and ensures accuracy in labeling.
- Automated Suggestions: Advanced algorithms can propose annotations, speeding up the process and ensuring consistency.
- Collaboration Capabilities: Multiple users can work on projects simultaneously, which promotes teamwork and increases efficiency.
- Quality Control Mechanisms: Features that allow for auditing and reviewing annotations help maintain high quality.
- Integration with Machine Learning Frameworks: Seamless integration with popular ML frameworks can enhance workflow efficiency.
The Advantages of Using Bounding Box Tools
Implementing bounding box annotation tools in your data annotation platform presents numerous benefits, including:
1. Enhanced Accuracy
High-quality annotations lead to improved accuracy in the models being trained. Precise labeling of objects allows algorithms to learn better and make reliable predictions.
2. Increased Efficiency
Automated tools can significantly reduce the time spent on data annotation. With features like automated object detection, bounding box tools can streamline the labeling process, enabling faster project turnaround times.
3. Scalability
As businesses grow, so does the volume of data they need to process. Using a robust bounding box annotation tool allows organizations to scale their data annotation efforts without compromising on quality.
4. Cost-Effectiveness
By optimizing the data annotation process, organizations can reduce operational costs associated with data preparation and model training.
5. Real-Time Collaboration
Teams can collaborate in real-time using cloud-based annotation tools, which fosters an environment for better project management and communication.
Use Cases for Bounding Box Annotation
There are numerous applications for bounding box annotation across various industries:
1. Autonomous Vehicles
In the automotive industry, bounding box annotations help identify and track objects in real time, critically assisting models that facilitate safe self-driving technologies.
2. Retail Analytics
In retail, businesses use bounding box annotations to analyze customer behavior and preferences by tracking consumer interactions with products.
3. Healthcare Imaging
In healthcare, annotated medical images help in the early diagnosis of diseases by identifying anomalies indicated by bounding boxes in X-rays, MRIs, and CT scans.
4. Agriculture Technology
Agricultural drones equipped with object detection algorithms can use bounding box annotations for effective crop monitoring and pest control strategies.
5. Security Surveillance
Security systems utilize bounding box annotations to identify and track moving objects, improving the efficacy of surveillance systems.
Implementing a Bounding Box Tool: Best Practices
To maximize the benefits of using bounding box annotation tools, consider the following best practices:
1. Define Clear Annotation Guidelines
Clear and comprehensive annotation guidelines help annotators understand the expectations and maintain consistency across annotations.
2. Invest in Quality Tools
Choose a data annotation platform that offers advanced bounding box tools with the features necessary for your specific needs—this investment will pay off in the long run.
3. Regularly Review Annotations
Implement a system for regular reviews and quality checks to ensure the integrity of the annotated data.
4. Train Your Team
Invest in training for your annotation team to enhance their understanding of the tools and maintain high standards in their work.
5. Leverage Automation
Make use of automated labeling suggestions to speed up the process, but ensure that human oversight is in place for quality assurance.
Conclusion
The integration of bounding box data annotation tools within your organization can lead to significant improvements in data quality and analysis efficiency. By embracing these innovative solutions, businesses like keylabs.ai can harness the power of machine learning more effectively, lead their markets, and unlock new opportunities for growth. As the need for accurate data annotation becomes increasingly critical with the rise of AI and machine learning technologies, investing in the right data annotation platform with comprehensive bounding box capabilities will undoubtedly position your business for future success.
In conclusion, the era of bounding box annotation is here, and it is a powerful ally in the realm of data science, providing a clear path for businesses to follow as they venture into the complexities of machine learning and artificial intelligence.